It starts innocently enough. A whimsical selfie, a doe-eyed version of yourself in a fantasy forest with fireflies and floating islands. Maybe you’re styled like a Studio Ghibli hero—somewhere between Totoro and your inner existential dread. You post it. People like it. You smile. What’s the harm, right?
But behind that dreamy image lies a growing infrastructure problem. In March 2025, OpenAI CEO Sam Altman wrote candidly on X, “It’s super fun seeing people love images in ChatGPT, but our GPUs are melting.” The company had just released its image generation feature to free-tier users, and the Studio Ghibli-style portraits—gentle, nostalgic, instantly shareable—had gone viral.
The demand was so overwhelming that OpenAI was forced to cap image generations at three per day per user, citing infrastructure stress. Altman followed up days later with an exhausted plea: “Can y’all please chill on generating images, this is insane. Our team needs sleep.”
But why would a simple animated selfie bring one of the world’s most advanced AI systems to its knees?
Image Generation vs. Text: A Resource Gap
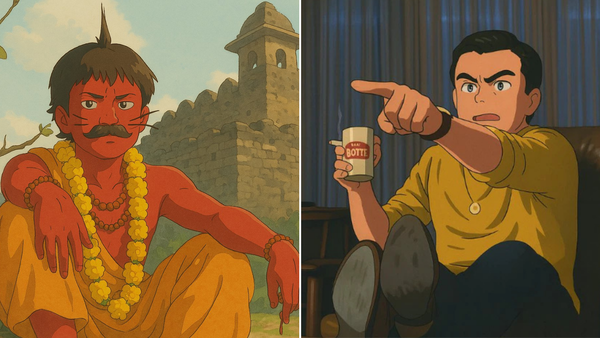
The answer lies in the massive energy and computational demands of generative AI—especially when it comes to images. A single AI-generated image requires roughly 1 trillion floating point operations (FLOPs) to create. For comparison, a typical text response from a language model uses around 100 billion FLOPs. In other words, generating one image is about 10 times more compute-intensive than generating text.
Most of these operations are handled by GPUs (Graphics Processing Units), which are designed for parallel tasks like image rendering. But GPUs are power-hungry. A high-end AI accelerator can consume up to 700 watts under full load. Multiply that by thousands of GPUs in a data center running simultaneously, and you get a sense of the energy involved in mass image generation.
This isn’t just an abstract concern. Diffusion models—the AI systems used for high-quality image generation—require dozens of iterative refinement steps to turn noise into a detailed image. Each of those steps draws heavily on GPU resources. According to estimates from Stanford and Hugging Face, a single image generated using a diffusion model consumes approximately 2.5 watt-hours of energy for computation alone. With cooling and infrastructure overhead included (typically calculated using a Power Usage Effectiveness, or PUE, of 1.3), the total rises to 3.25 watt-hours per image.
That’s about the same as running a 60-watt lightbulb for 3.25 minutes—or charging a smartphone to 50%. It may seem trivial until you realize that millions of users were generating multiple images each, often just for fun or aesthetic experimentation.
From Data Centers to the Grid
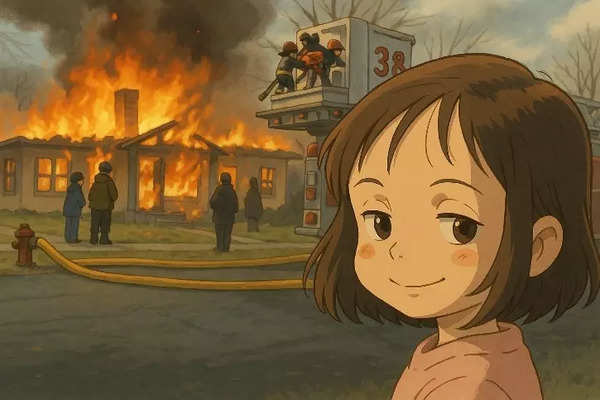
Amid the unprecedented rise in demand for the ‘Ghibli’ image generator feature by ChatGPT, OpenAI’s CEO, Sam Altman, took to his X account to request social media users to ‘chill out a bit’ as his team needs rest. Read more.
Each of those images is processed in massive data centers that house rows of GPUs in climate-controlled conditions. These facilities are not lightweight operations. Globally, data centers already account for 1–1.5% of total electricity consumption, and that number is rising quickly with the spread of generative AI.
Cooling systems are a major part of the problem. GPUs operating under sustained load generate considerable heat, requiring sophisticated liquid or air cooling systems. In areas like Arizona or Utah—where several AI and cloud providers operate—cooling can also involve evaporative water systems, drawing hundreds of thousands of gallons per day. In some cases, AI data centers have been projected to consume tens of millions of gallons of freshwater per year, raising concerns in drought-prone regions.
These environmental pressures become more acute when trends go viral. What might seem like a fun, personal use of AI scales rapidly to global infrastructure demand. A single image may not matter. Billions of them do.
Why Ghibli Hit Harder
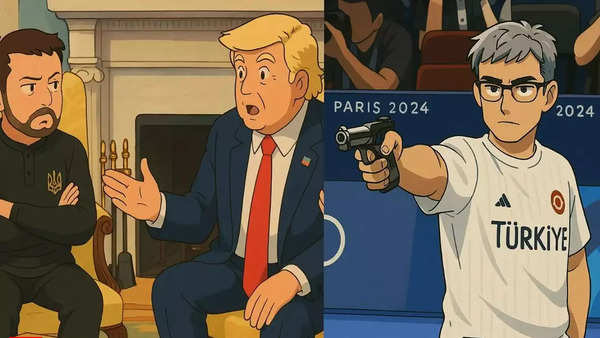
The Ghibli-style trend hit a perfect cultural nerve. The output was undeniably charming: less uncanny than previous AI portraits, rich with nostalgia, and globally recognizable. People didn’t just generate one—they experimented, tweaked, shared. They ran photos of pets, family members, historical figures, and even politicians through the filter.
What made this trend especially potent was its visual fidelity. These weren’t just stylized approximations—they closely resembled actual frames from Studio Ghibli films, evoking deep emotional and aesthetic appeal. The quality and shareability of the outputs supercharged engagement, fueling an exponential spike in demand.
Environmental Implications: A Broader Pattern
The Ghibli image boom is a microcosm of a much larger issue: the unseen environmental cost of digital trends.
While AI art is just one sliver of digital consumption, it represents a broader shift. The cloud—often thought of as abstract and intangible—is in fact a sprawling network of physical infrastructure, most of which still runs on nonrenewable energy. From video streaming to blockchain mining, our digital habits are increasingly powered by a real-world grid, with real-world consequences.
And while some tech companies are investing in renewable energy and efficiency gains, many AI models are still trained and run in facilities connected to traditional power sources. Even when companies purchase carbon offsets or renewable energy credits, the net energy demand of AI continues to grow faster than sustainability improvements can catch up.
What Can Be Done?
OpenAI and other providers are actively optimizing their models and infrastructure. Reducing inference time, improving GPU efficiency, and scaling with newer, more efficient hardware are all part of the response. But these are technical solutions to a cultural issue.
The key challenge is managing expectations and awareness. AI image generation is no longer a niche feature—it’s a mass-market product. And like any popular product, it needs to be used thoughtfully. While there’s no need to stop using AI art tools entirely, treating them as digital luxuries rather than casual toys may help shift behavior toward sustainability.
Public education can also play a role. If platforms displayed a small energy-use estimate per image—similar to nutrition labels on food—it might help users understand the real-world cost of their creative choices. And just as consumers have embraced slow fashion or low-waste living, there may be room for “slow content” in the AI age.
Final Thought
AI-generated portraits, especially in beloved styles like Studio Ghibli’s, offer a fun and often beautiful way to express ourselves. But each one is the product of an immense chain of compute, energy, and infrastructure.
Understanding this doesn’t mean we have to abandon creativity or fun—it simply means using these tools with intention. Because while the images may be imaginary, their impact is very real.